NVIDIA is making revenue growth out of AI models gaining significant momentum and global attention.
In its Fiscal 2024 report, the company earned quarterly revenue of $22.1 billion, a jump of 265% from 2023. It earned $18.4 billion of quarterly Data Center revenue, a boost of 409% from 2023. The full-year revenue of NVIDIA is $60.9 billion for the fiscal year 2024.
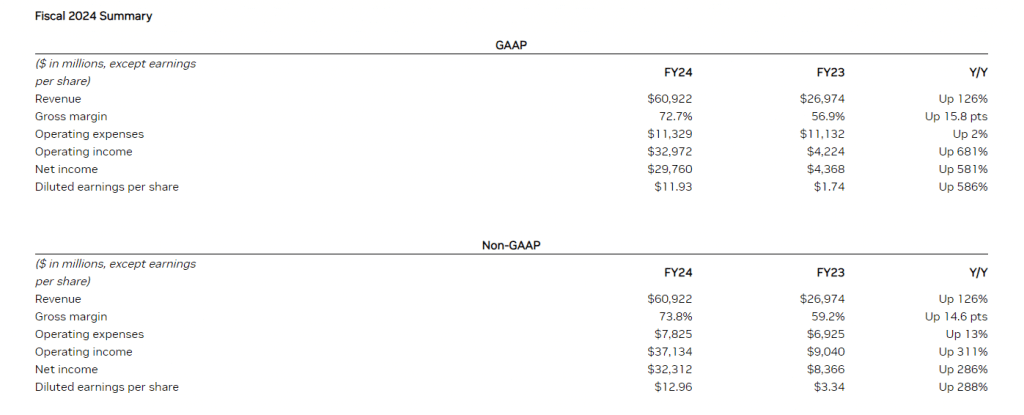
Source: NVIDIA
Since the GPUs-intensive generative models depend on powerful semiconductors to process large amounts of training data for AI algorithms, no wonder why NVIDIA is seen as a dominating player in the AI race.
I also see the company’s unstoppable revenue growth based on the future rise of supercomputers, increasing demand for generative AI, and of course the new shift in the world of Metaverse and XR.
The world I see few years down the line will be overtly dominated by superintelligent AI machines. These AI machines will require powerful semiconductors to perform as expected. Meanwhile, AI-led automation has got the world thinking toward AI machines as a money-spinning investment for companies like OpenAI, Microsoft, and Google.
What Does NVIDIA Mainly Do For AI?
NVIDIA is one of the most instrumental players in the field of ai development by designing powerful GPUs (graphics processing units) suitable for huge computational requirements when training large AI models.
Since GPUs do an excellent job in parallel processing, they can efficiently address complex tasks, such as massive computations in training large AI models. These GPUs keep innovating to increase their inherent performance and efficiency level to serve AI workloads effectively.
Also Read – Generative AI in Creative Works
Not only the hardware front, NVIDIA also does a great job in building different types of software to complement their GPUs and streamline AI workloads. For example, its software libraries called Deep Learning Frameworks encompass the necessary tools and functions to build and train deep learning AI models.
An example of such a suite is NVIDIA CUDA-X AI. It includes deep learning frameworks such as cuDNN and TensorRT. Functionalities offered by the suite are for training, optimization, and deployment of deep learning models on NVIDIA GPUs.
Other tools built by NVIDIA are Model Optimization tools like NVIDIA TensorRT, and Scalability Tools like NCCL (NVIDIA Collective Communications Libraries).
To put simply, the role of NVIDIA in AI is to provide essential hardware and software muscle power, enabling AI researchers and developers to build and train ever more complex AI models. It designs robust GPUs to streamline the massive computations required in AI workloads.
How Will NVIDIA Address Scalability And Performance Challenges In The Anticipated Rise of Superintelligent AI Machines Or Emerging Technologies Like Quantum Computing?
As far as my understanding is concerned, NVIDIA will have a substantial role in addressing the scalability and performance challenges posed by AI models. This would involve NVIDIA’s persistent refinement in its core technologies together with ensuring that it fully acclimatizes to the fast-changing landscape of AI hardware.
We must keep this in mind that NVIDIA does an excellent job in designing a powerful GPU (Graphics Processor Unit) to streamline massive computations involved in AI workloads.
As far as quantum computing is concerned, NVIDIA will explore every option to ensure its relevant coexistence with the advancements in quantum computing, as well as innovating its hardware and software (i.e. GPU performance) and building efficient AI frameworks to handle the growing challenges of AI models.
So, the point is, with the pace of development made in quantum and neuromorphic computing, NVIDIA may integrate these advancements for solutions to build a large-scale AI ecosystem consisting of various AI technologies and tools to seamlessly achieve many coveted goals, including developing open-source AI tools and frameworks. That’s my projection right now.
How Would NVIDIA Contribute To Building A More Comprehensive AI Ecosystem?
The concept of having a full-fledged AI ecosystem simply means an ecosystem consisting of a variety of AI technologies and tools to seamlessly work together to achieve different sets of goals.
The contribution of NVIDIA in building a comprehensive AI ecosystem is not just limited to building powerful hardware. It goes beyond that. It will contribute to developing open-source AI tools and frameworks. Moreover, it will explore the hardware capabilities of other software companies and research institutions ensuring that they can be utilized with other AI technologies. Further, the company will take a stand in advocating for open standards.
What Does It Mean By Advocating For Open Standards?
When we talk about ai, the term, ‘open standards’ means the manner in which different AI models and tools communicate and exchange data. It appears like building a common language for ai components (i.e. a broad range of elements working together to enable AI functionality.).
NVIDIA will advocate for open standards in terms of different things. For example, it will advocate for building wider collaboration and adoption of open-source ai frameworks and tools. They would foster open communication with other AI companies and channels to collaborate on open standard development.
In brief, advocating for open standards means NVIDIA’s role in strengthening the overall AI ecosystem, and enhancing innovation for everyone.
Please note that AI components here simply mean the overall ecosystem of tools and models seamlessly working together. It also includes ai models and other elements, like deep learning frameworks, optimization tools, scalability tools, etc.)
Conclusion
NVIDIA has a strong footprint in AI race today. Though it would be hasty to say its growth is unstoppable, there is no doubt that it has registered its impressive revenue growth. Reportedly, the company has increased its revenue growth attributed to its data center business catering to AI workloads. The impressive contributions of the company have also brought its competitors to the forefront of AI revenue growth.